
#Cerebro elasticsearch how to
This first article - ELK-MS - part I : setup the cluster in this serie presents the ELK-MS stack and how to set up a test cluster using the niceideas ELK-MS package. I am writing a serie of two articles to present this stack and why it's cool. Today this state of the art ELK-MS - for ElasticSearch/Logstash/Kibana - Mesos/Spark stack performs amazingly and I believe it to be a really lightweight, efficient, low latency and performing alternative to a plain old Hadoop Stack. Now of course we were initially still considering a Hadoop stack for the single purpose of using YARN as resource management layer for Spark. We are using ElasticSearch as key storage backend and Apache Spark as processing backend. We considered a whole lot of different approaches, but eventually, and contrary to what I expected first, we didn't settle for a standard Hadoop stack.
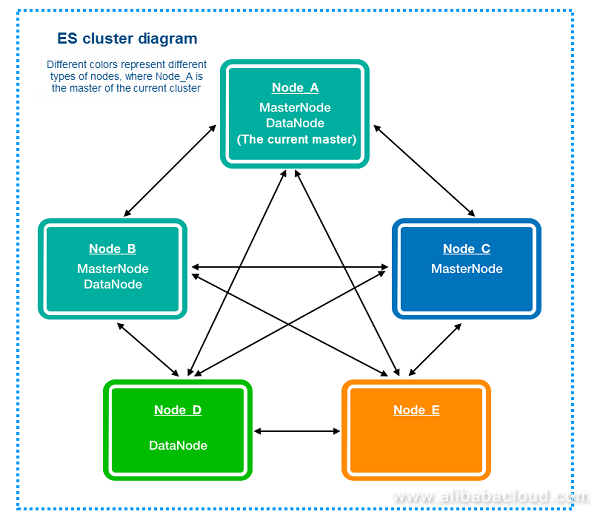
Historically, these heavy computations were taking a whole lot of different forms, mostly custom computation scripts in python or else using RDBMS databases to store data and results.Ī few years ago, we started to hit the limits of what we were able to achieve using traditional architectures and had to move both our storage and processing layers to NoSQL / Big Data technologies. In my current company, we implement heavy Data Analytics algorithms and use cases for our customers. The Spark 2.2.0 Dynamic allocation system is now perfectly compatible with the way ES-Hadoop 6.x enforces data locality optimization and everything works just as expected. With ElasticSearch 6.x, and ES-Hadoop 6.x, the game changes a little. Edited : I was using ES 5.0.0 with Spark 2.2.0 at the time of writing the initial version of this article.
